基于微博数据的用户画像与精准营销
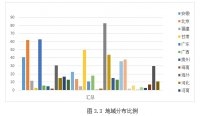
基于微博数据的用户画像与精准营销(任务书,开题报告,论文14000字)
摘 要
随着互联网和云计算技术的迅猛发展,大数据时代已经悄然来临。在大数据时代,使用相关的技术手段对微博用户的数据进行采集和分析,建立用户画像的模型,便于更好的了解用户的兴趣偏好以及行为偏好,使精准营销成为了可能。本文着力研究的是在利用微博的话题聚合来建立用户画像,所以本文是建立在基于微博话题的背景下建立用户画像,通过文献研究法,爬取数据法以及数据分析法,分析微博用户在某一话题下某一个人或者某一群人的用户信息,并且用这些用户信息给用户贴上标签,通过这些标签,我们就能准确的为用户建立相关的用户画像模型。企业就能够通过我们建立的用户画像模型来对目标人群进行分类管理,为他们制定良好的个性化推荐与个性化服务,进而加快企业精准营销的进度。
关键词:大数据;基于微博;用户画像;精准营销
Abstract
With the rapid development of Internet and cloud computing technology, the era of big data has quietly come. In the era of big data, relevant technical means are used to collect and analyze the data of weibo users and establish the model of user portrait, so as to better understand the interests and behavioral preferences of users and make precision marketing possible. Focus on the research of this article is on the issue of using weibo polymerization to build user portrait, so this article is based on based on weibo topics under the background of the user, through literature research, crawl data and data analysis, analysis of weibo users in a certain topic one person or a group of people under the user information, and labeled with the user information to the user, through these labels, we can accurate portraits related user model is established for the user. Enterprises can classify and manage target groups through the user portrait model established by us, develop good personalized recommendations and personalized services for them, and then accelerate the progress of enterprise precision marketing. [版权所有:http://DOC163.com]
Keywords:Big data;Based on weibo;Personas;Precision marketing
[来源:http://Doc163.com]
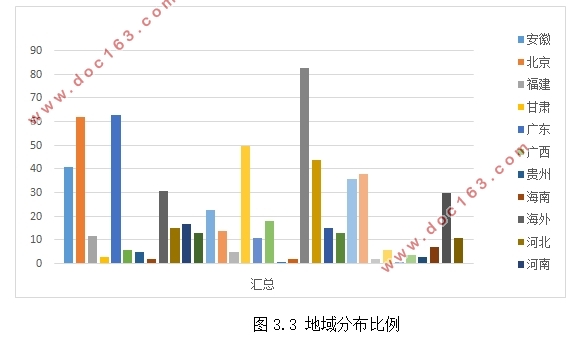
目录
摘 要 I
Abstract II
1绪论 1
1.1研究背景及意义 1
1.2相关研究综述 1
1.2.1大数据研究综述 1
1.2.2用户画像构建方法研究综述 2
1.2.3精准营销研究综述 3
1.3本文研究内容 3
1.4研究方法与研究思路 3
2基于微博数据的用户画像 5
2.1用户画像的概述 5
2.2用户画像的构建方法 5
2.2.1基于用户行为的画像方法 5
2.2.2基于用户兴趣偏好的画像方法 6
2.2.3基于主题的用户画像方法 6
2.2.4基于人格特性和用户情绪的画像方法 6 [资料来源:http://doc163.com]
2.3用户画像的数据源获取 6
2.4用户画像标签建模 7
3基于微博话题的用户画像模型建立 9
3.1 数据源获取 9
3.2 用户画像构建 10
3.3 微博精准营销 13
4 用户画像在精准营销中的应用 15
4.1 发现营销的目标人群 15
4.2 更加准确迅速的掌握用户的动向 16
4.3 将潜在用户转化为目标用户 16
4.4 进行更加精确的推送 17
5 总结与展望 18
参考文献 19
致谢 20