基于静息态fMRI的脑功能网络分割
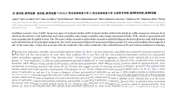
基于静息态fMRI的脑功能网络分割(任务书,开题报告,论文23000字)
摘要
不论是构建大脑功能模型、探索人脑连接组,还是研究疾病对功能连接的影响,一个至关重要的步骤为定义功能网络节点。将全脑体素作为功能网络的节点会导致维数灾难(Curse of Dimensionality),对计算性能要求过高,并且易受噪声干扰。此外,全脑体素水平的功能网络中存在着大量的冗余数据,这也将导致后续网络分析变得棘手。因此,将体素水平的功能网络进行分割尤为重要。脑网络分割也就是将大脑分为一组互不相交且空间连续的区域,每一区域也称为一个脑区,每一脑区对应着ROI(Region of Interest)水平功能网络的一个顶点。
现有方法主要分为三类:手动标注、基于解剖学模版的分割、静息态功能性磁共振(resting-state functional Magnetic Resonance Imaging,R-fMRI)数据驱动的分割。手动标注需要较多的先验知识,且容易产生错误,使得分析结果产生偏差。基于解剖学模版的分割优于手动标注,但是已有研究表明,解剖结构的脑区划分与功能分区并不一致,并不能完全反映大脑的内在功能组织。因此,本文使用数据驱动的聚类算法进行功能网络分割。本文的方法首先对每个被试的数据在体素水平上构建无向图模型,根据全脑体素R-fMRI时间序列的相似性计算邻接矩阵。然后基于提出的空间约束N-Cut(Normalized Cut)谱聚类算法对每个被试邻接矩阵进行分割,得到个体水平的分割结果。最后结合多个个体水平分的割结果计算群体水平的邻接矩阵,并进行N-Cut谱聚类,得到群体水平的脑功能网络分割结果。 [资料来源:http://www.doc163.com]
与两种基于大脑结构信息得到的解剖学脑图谱以及另一种数据驱动的分割方法在五种评价指标上的对比结果表明,本文的分割方法能够更加准确地进行大脑功能划分,使每一脑区内部具有较高的功能一致性,不同脑区之间具有较高的功能差异性。同时,年龄预测任务上较高的准确率表明了本文方法的分割结果构建的功能网络能够较好地捕获个体的功能连接差异,能够为基于静息态fMRI的人脑认知过程研究以及精神疾病研究提供有效的支持。
关键词:大脑分割;谱聚类;功能性磁共振成像;静息态;功能连接
Abstract
Whether building a function model of brain, exploring a human connectome project, or studying the effects of disease on functional connectivity, a crucial step is to define functional network nodes.Using voxel in imaging volume of brain as a node of a functional network leads to curse of dimensionality, and it is too demanding on computational resources and sensitive to noise. In addition, the functional network at voxel level has a large amount of redundant data, which will alsolead to subsequent network analysis becoming tricky. Thus, parcellating the brain is crucial. What we should do is parcellating the brain into a set of mutually disjoint and spatially continuous regions. Each region is also called a brain region. Each brain region corresponds to a vertex of the functional network at ROI (Region of Interest) level.
[资料来源:Doc163.com]
Existing methods can be divided into three categories: manual labeling, anatomical atlas based parcellation, and R-fMRI(resting-state functional magnetic resonance imaging) data driven parcellation.Manual labeling method requires more prior knowledge in neuroanatomical and is prone to errors, which leads to bias in results. Anatomical atlas based parcellation is usually better than manual labeling, but previous researches have shown that the brain region derivedfrom anatomical information is inconsistent with functional parcellation, andcan’t correctly reflect the intrinsic functional organization of the brain. Thus, we parcellating the brain based on data driven clustering algorithm. We model the voxels of whole brain as undirected graph, and calculate the adjacent matrix using the similarity of time courses of voxel pair in whole brain. Then, based on proposed spatial constraint N-Cut (Normalized Cut) spectral clustering algorithm, we parcellate the adjacent matrix for each subject, and get individual-level parcellations. Finally, we calculate the group-level adjacency matrix by combing multiple individual-level parcellations. We perform the N-Cut on the group-level adjacency matrix and get the group-level parcellations. [来源:http://www.doc163.com]
Comparison with two anatomical atlas based on brain structure information and another data-driven parcellating method on five evaluation criteria indicate that proposed method can parcellate the brain more precisely and maintain higher functional homogeneity in a region as well as make higher functional difference between brain regions. At the same time, the higher accuracy in aging prediction task indicates that the functional network of brain constructed by proposed method can capture the functional connectivity difference between different subjects more precisely, and can provide effective support for cognitive or mental illness research based on R-fMRI.
Keywords: brain parcellation; spectral clustering; fMRI; resting state; functional connectivity
目录
第1章绪论 1
1.1 研究的目的与意义 1
1.2 国内外研究现状 2
1.3 论文内容安排 3
第2章静息态fMRI原理 4 [资料来源:http://doc163.com]
2.1 功能性磁共振成像 4
2.2 任务态与静息态 5
2.3 静息态功能网络 6
2.4 静息态功能网络的网络分析 7
2.4.1 网络分析 7
2.4.2 大脑功能网络的特性 8
2.5 本章小结 9
第3章基于谱聚类的脑功能网络分割原理 11
3.1 空间约束N-Cut谱聚类原理 11
3.1.1 全脑体素无向图模型 11
3.1.2 N-Cut谱聚类 11
3.1.3 空间约束 13
3.2 基于空间约束N-Cut的脑功能网络分割 15
3.3 本章小结 16
第4章脑功能网络分割的实现与结果 17
4.1 静息态fMRI数据集 17
4.2 实现与计算 17
4.3 静息态fMRI数据预处理 17
4.4 脑功能网络分割结果 18
4.5 本章小结 22
第5章脑功能网络分割结果评估 23
[资料来源:http://Doc163.com]
5.1 评价指标 23
5.1.1 再现性 23
5.1.2 聚类质量 24
5.1.3 年龄预测任务 25
5.2 分割结果性能评估 26
5.3 本章小结 29
第6章总结与展望 31
参考文献 33
致谢 39 [版权所有:http://DOC163.com]