基于拉普拉斯特征映射的滚动轴承故障状态识别方法研究
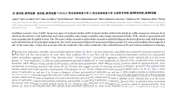
资料介绍:
基于拉普拉斯特征映射的滚动轴承故障状态识别方法研究(任务书,开题报告,外文翻译,论文14000字)
摘要
滚动轴承是旋转机械中经常发生故障的部件,其工作状态影响着整个旋转机械的运行状态,所以对其进行诊断就尤为重要。滚动轴承故障诊断主要包括采集振动信号、特征提取、模式分类三部分,其中特征提取是最关键的环节。滚动轴承的故障信号,往往具有高维,非线性,非高斯分布等特征。拉普拉斯特征映射算法(Laplacian Eigenmaps,LE)是一种聚类性较好的流形学习算法,且具有处理高维非线性数据的能力,所以将LE算法应用于滚动轴承故障诊断,并在MATLAB平台上进行一些比较实验来验证其有效性。
以滚动轴承作为研究对象,将LE算法应用于滚动轴承的故障状态识别,建立基于LE的滚动轴承故障状态识别模型。该模型利用LE算法,直接从原始振动信号构建的高维数据空间中,提取出低维流形特征,并将其输入K近邻分类器中进行分类。为了进一步验证LE算法的有效性,将LE算法与核主成分分析算法(KPCA)、核判别分析算法(KDA)进行对比试验。主要有以下四组试验:四种故障类型识别、五种故障损伤程度识别、不同负载下故障的识别、七种随机故障的识别。在这几组实验中,LE算法始终表现出了良好的聚类性以及较高的故障识别准确率,充分验证了LE算法对于滚动轴承故障识别的有效性。最后,研究了LE算法的特征维数和近邻点个数对滚动轴承故障识别精度的影响。
[资料来源:http://doc163.com]
关键词:滚动轴承;故障诊断;特征提取;拉普拉斯特征映射
Abstract
Rolling bearingisa component which often breaks down in rotating machinery, and its working state affects the running state of the whole rotating machinery.So it is very important to diagnose it. Fault diagnosis mainly includes three parts, such as collecting vibration signal, feature extraction and pattern classification, among which feature extraction is the most critical link. The fault signal of rolling bearing often has the characteristics of high dimensional, nonlinear, non-Gaussian distribution and so on. Laplace feature mapping algorithm (Laplacian Eigenmaps, LE) is a well-clustering manifold learning algorithm with the ability to handle high-dimensional nonlinear data, so the LE algorithm is applied to the fault diagnosis of rolling bearings, and some comparative experiments are carried out on the MATLAB platform to verify its effectiveness. Taking rolling bearings as the research object, the LE algorithm is applied to the fault state identification of rolling bearings, and the fault state identification model of rolling bearing based on LE is established. Using LE algorithm, the model extracts the low dimensional manifold features directly from the high-dimensional data space constructed by the original vibration signal, and classifies them into the K neighbor classifier. In order to further verify the validity of LE algorithm, the LE algorithm is compared with the kernel principal component analysis algorithm (KPCA) and the kernel discriminant analysis algorithm (KDA). There are four groups of tests: four fault type identification, five fault damage degree identification, fault identification under different loads, and identification of seven kinds of random faults. In these groups of experiments, LE algorithm always shows good clustering and high fault recognition accuracy, and fully verifies the effectiveness of LE algorithm for the fault identification of rolling bearings. Finally, the influence of the characteristic dimension and the number of near-neighbor points of LE algorithm on the fault recognition accuracy of rolling bearing is studied.
[资料来源:http://www.doc163.com]
Key Words:Rolling bearing ; Fault diagnosis ; Feature extraction ; Laplacian Eigenmap
研究的基本内容、目标、拟采用的技术方案及措施
流形学习方法在故障诊断领域内已得到了广泛地应用。它不仅可以被用作用于非平稳故障信号的有效噪声降低的方法,但还可以提取用于状态监测和趋势预测。在故障诊断领域流形学习方法的应用非常广泛,值得深入研究。本文以流形学习理论以及拉普拉斯特征映射为基础,针对旋转故障诊断中存在的问题进行研究。本文的内容安排如下:
第一章,介绍旋转轴承故障状态识别的研究背景及意义。本文讨论了滚动轴承诊断面临的问题以及传统故障诊断方法的不足。简要介绍了流形学习中的拉普拉斯特映射及其在故障状态识别中的应用前景。
第二章介绍了流形学习方法和拉普拉斯特征映射方法,并阐述了该算法的理论基础和用法。
第三章,基于拉普拉斯特征映射的诊断研究。使用流形学习中的拉普拉斯特征映射算法(LE)对滚动故障信号进行诊断,在故障信号中的高位数据信号中提取出具有代表性的低维流形特征。
第四章,旋转机械状态评估。对使用基于LE算法的故障诊断方法的结果与传统算法诊断进行对比分析,分析故障原因,诊断故障结果。 [资料来源:http://www.doc163.com]
第五章总结了研究工作和全文的结果,并期待着在旋转机械故障诊断方法的进一步研究方向。
第2章拉普拉斯特征映射算法及基于该算法
的智能诊断模型
目录
摘要 I
Abstract II
第1章绪论 1
1.1 研究目的及意义 1
1.2滚动轴承故障诊断技术的研究现状状 1
1.3流形学习与拉普拉斯特征映射在机械故障诊断领域的研究现状 2
1.4研究(设计)的基本内容、目标、拟采用的技术方案及措施 3
第二章拉普拉斯特征映射算法及基于该算法的智能诊断模型 4
2.1流形学习方法与分类介绍 4
2. 2 LE 算法基本原理 5
2.3其它几种流形学习算法 6
2.3.1核主成分分析算法 6
2.3.2核判别分析算法 7
2.4基于LE算法的故障诊断模型 8
第三章故障状态识别实验 9
3.1实验装置 9 [资料来源:http://www.doc163.com]
3.2三种诊断方法实验分析 10
3.2.1 实验方案设计 10
3.2.2 滚动轴承四种故障类型的识别 10
3.2.3滚动轴承五种损伤程度的识别 13
3.2.4滚动轴承在轻微故障程度下五种负载的故障识别 14
3.2.5 七种随机故障状态的识别 17
3.2.6不同样本下滚动轴承的故障识别精度 20
3.2.7三种算法在最高故障识别率时的参数设置 21
3.2.8特征维数d与近邻点个数K对识别精度的影响 22
第四章总结与展望 23
4.1总结 23
4.2展望 23
参考文献 24
致谢 26 [版权所有:http://DOC163.com]