基于大数据的可用停车位预测方法研究
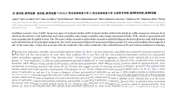
资料介绍:
基于大数据的可用停车位预测方法研究(任务书,开题报告,论文14000字)
摘要
经济迅猛发展,大众出行方式日益多样,汽车数量大幅增长,停车位不足成为交通难题。可用停车位预测是城市智能交通中停车引导系统的关键技术,有助于驾驶员高效地选择停车场地以及规划行车路线。针对该问题,国内外现有的预测方法研究重点在于短期预测,但对未来一段时间内停车场可用停车位变化趋势的预测远比实时获取可用停车位数量重要。因此需要建立短期、及长期预测的可用停车位预测模型。
本文的数据来源为武汉市某医院地下停车场的停车数据,基于大数据平台技术,对可用停车位时间序列进行数据特征的统计与分析,提出基于长短期记忆(Long Short-Term Memory, LSTM)神经网络的可用停车位单步和多步预测模型,并进行与传统模型的对比,从而表明所用模型在预测性能上的优势。探讨多步预测法常见的两种策略,分析两者的理论特征及各自优缺点,提出实际应用中的适用场景,为多步预测方法研究提供了理论支持。
基于可用停车位数据进行仿真实验,实验结果表明基于LSTM神经网络的可用停车位单步预测模型在预测精度和稳定性上表现更优,在多步预测上也体现了较好的预测性能。
关键词:可用停车位;大数据平台;长短期记忆;神经网络
[资料来源:www.doc163.com]
Abstract
With the continuous development of urban construction in China, residents’ demand for travel has increased and the number of urban vehicles has increased dramatically. The shortage of parking spaces has become a major problem for urban transport.Predicting the available parking space technology is the key technology of intelligent parking guidance system in urban intelligent transportation, which plays an important role for drivers to efficiently and reasonably select parking lots and plan their driving routes.With regard to this issue, the existing forecasting methods at home and abroad focus on short-term forecasting, but it is more important to predict the changing trend of parking spaces available in parking lots than in real-time.Therefore, it is necessary to establish a parking prediction model with single-step and multi-step prediction functions.
The data source of this article is the parking data of an underground parking lot of a hospital in Wuhan City. Based on the big data platform technology, the data characteristics of available parking space time series are analyzed.A single-step and multi-step prediction model of available parking spaces based on Long Short-Term Memory (LSTM) neural network is proposed and compared with the traditional model, which validated the advantages of the model used in prediction performance.The multi-step prediction scenarios of the iterative method and the direct method are discussed. By analyzing the theoretical characteristics of the two methods and their respective advantages and disadvantages, the applicable scenarios in the practical application are put forward, which provides certain theoretical support for the research of multi-step prediction methods. [资料来源:http://doc163.com]
Based on the available parking space data, simulation experiments are performed. The experimental results show that the LSTM neural network-based parking space single-step prediction model performs better in prediction accuracy and stability, and it also shows better prediction performance in multi-step prediction.
Key Words:Available parking space;Big data platform;Long Short-Term Memory;Neural Networks
目录
第1章绪论 1
1.1 研究背景 1
1.2 研究意义 1
1.3 研究现状 2
1.3.1短期预测 2
1.3.2 长期预测 3
1.4 研究内容 3
1.5 论文结构 4
第2章数据特征分析 5
2.1 数据挖掘工具 5
2.2数据预处理 5
2.2.1 数据源介绍 5
2.2.2 数据筛选 6
2.3 数据基本特征分析 7 [资料来源:http://www.doc163.com]
2.3.1 数据规律性 7
2.3.2 数据波动性 7
2.4数据的周期性分析 8
2.5数据的混沌性分析 9
第3章可用停车位单步预测方法研究 11
3.1 LSTM神经网络 11
3.1.1 LSTM神经网络的基本结构 11
3.1.2 LSTM神经网络的关键参量 13
3.1.3 LSTM神经网络预测算法 14
3.2基于LSTM的可用停车位单步预测模型 16
3.2.1 基于LSTM的单步预测模型构建 16
3.2.2 模型预测性能的评价方案 16
3.3基于LSTM的可用停车位单步预测模型实现 17
3.3.1 单步预测模型的实现 17
3.3.2 单步预测实验结果分析 18
3.3.3 影响模型性能的因素 20
第4章可用停车位多步预测方法研究 22
4.1 多步预测方法原理 22
4.2基于LSTM的可用停车位多步预测模型 22 [来源:http://Doc163.com]
4.3 多步预测实验结果分析 23
第5章总结与展望 25
5.1 本文研究内容 25
5.2 未来工作展望 25
参考文献 26
致谢 28 [资料来源:Doc163.com]